Gaurav Bhardwaj
Assistant Professor
University of Washington
Talk Information
Computational Empowerment in Peptide Science
18 June 2025, 03:30pm - 03:45pm, in the Pacific Jewel Ballroom
L47 – Generative Deep Learning for Accurate de novo Design of Macrocyclic Peptides
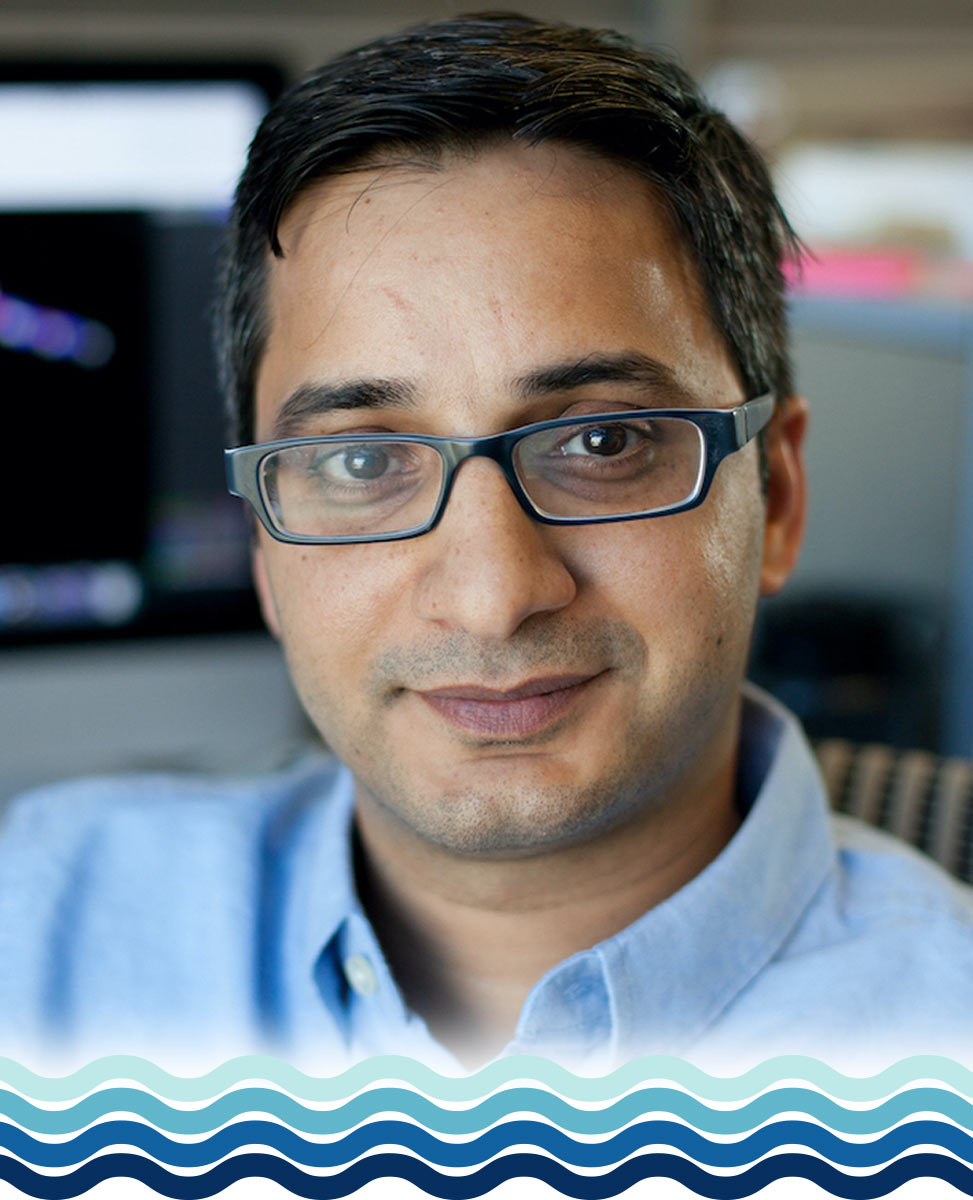
Professor Gaurav Bhardwaj is an esteemed researcher specializing in computational peptide design, currently serving as an Assistant Professor in the Department of Medicinal Chemistry at the University of Washington's School of Pharmacy. He is also affiliated with the Institute for Protein Design, contributing significantly to the field of peptide-based therapeutics.
Academic Background
Professor Bhardwaj earned his Bachelor of Technology in Biotechnology from GGS Indraprastha University in Delhi. He then pursued a Ph.D. in Integrative Biosciences at Pennsylvania State University, where he developed computational methods for studying the evolution of highly divergent protein families. Following his doctoral studies, he completed postdoctoral research with Dr. Kit Lam at the University of California, Davis, and Dr. David Baker at the University of Washington, focusing on the computational design of hyperstable constrained peptides and macrocycles with atomic-level accuracy.
Research Focus
Professor Bhardwaj's research centers on developing computational and experimental tools for the high-throughput design of peptide-based therapeutics. His lab aims to establish a comprehensive set of design principles for creating peptides with enhanced membrane permeability, oral bioavailability, and the ability to traverse the blood-brain barrier. The lab's work addresses a broad range of disease-related molecular targets, including those associated with antibiotic resistance, chronic pain, and neurodegenerative disorders.
Notable Publications
Accurate de novo design of hyperstable constrained peptides, Nature, 2016.
Comprehensive computational design of ordered peptide macrocycles, Science, 2017.
Accurate de novo design of membrane-traversing macrocycles, Cell, 2022.
Design and structural validation of peptide–drug conjugate ligands of the kappa-opioid receptor, Nature Communications, 2023.
Expansive discovery of chemically diverse structured macrocyclic oligoamides, Science, 2024.
Professional Engagements
In addition to his academic roles, Professor Bhardwaj is the scientific co-founder of Vilya, a biotechnology company focused on peptide drug design and discovery. His lab actively collaborates with various departments and research institutes, including the Molecular Engineering & Sciences Institute and the Institute for Protein Design at the University of Washington. Through these collaborations, he continues to advance the field of peptide therapeutics, contributing to the development of innovative treatments for a range of diseases.
Generative Deep Learning for Accurate de Novo Design of Macrocyclic Peptides
Department of Medicinal Chemistry, University of Washington, Seattle; Institute for Protein Design, University of Washington, Seattle
The development of deep learning methods, such as AlphaFold and RoseTTAFold, has considerably improved the accuracy of structure prediction and design of larger proteins1,2. However, these deep learning methods typically do not apply or work well for small peptides with non-canonical amino acids. We previously described state-of-the-art “physics-based” methods for accurately designing hyperstable constrained peptides, macrocycles3,4, and membrane-traversing macrocycles5. To address the compute-related and functional limitations of those previous physics-based methods, we recently developed deep learning (DL) methods, AFCycDesign6 and RFpeptides7, for highly accurate structure prediction, sequence design, and de novo generation of macrocyclic peptides.
These new DL tools outperform the traditional methods in their speed, accuracy, and overall success rates. We recently leveraged RFpeptides to successfully design macrocyclic peptide binders for multiple therapeutically-relevant protein targets, including targets with no available crystal or CryoEM structures7. In contrast to library-based approaches, we tested only 20 or fewer designs against each target and yet identified macrocycles with nanomolar (< 10 nM) binding affinities toward their targets. X-ray crystal structures of this protein-bound macrocycle match very closely (RMSD < 1.5 Å) with their computational models, confirming the designed structure and binding mode. In addition to applying these generative tools for designing new antibiotics, antivirals, and degraders for rare pediatric cancer targets, we are also expanding these DL methods to design precisely controlled oligomeric assemblies and incorporate the vast chemical diversity of non-canonical amino acids. Together, these new DL-guided methods enable custom and rapid design of peptides for diverse structures and functions.
- Jumper, J. et al. Highly accurate protein structure prediction with AlphaFold. Nature 596, 583–589 (2021).
- Baek, M. et al. Accurate prediction of protein structures and interactions using a three-track neural network. Science 373, 871–876 (2021).
- Bhardwaj, G. et al. Accurate de novo design of hyperstable constrained peptides. Nature 538, 329–335 (2016).
- Hosseinzadeh, P. et al. Comprehensive computational design of ordered peptide macrocycles. Science 358, 1461–1466 (2017).
- Bhardwaj, G. et al. Accurate de novo design of membrane-traversing macrocycles. Cell (2022) doi:10.1016/j.cell.2022.07.019.
- Rettie, S. A. et al. Cyclic peptide structure prediction and design using AlphaFold. bioRxiv (2023) doi:10.1101/2023.02.25.529956.
- Rettie, S. A. et al. Accurate de novo design of high-affinity protein binding macrocycles using deep learning. bioRxiv (2024) doi:10.1101/2024.11.18.622547.