Yu-Shan Lin
Professor - Department Chair
Tufts University
Talk Information
Session 9: Computational Empowerment in Peptide Science
18 June 2025, 02:50pm - 03:15pm, in the Pacific Jewel Ballroom
L46 – Integrating Molecular Dynamics Simulation & Machine Learning for Cyclic Peptide Structure Prediction
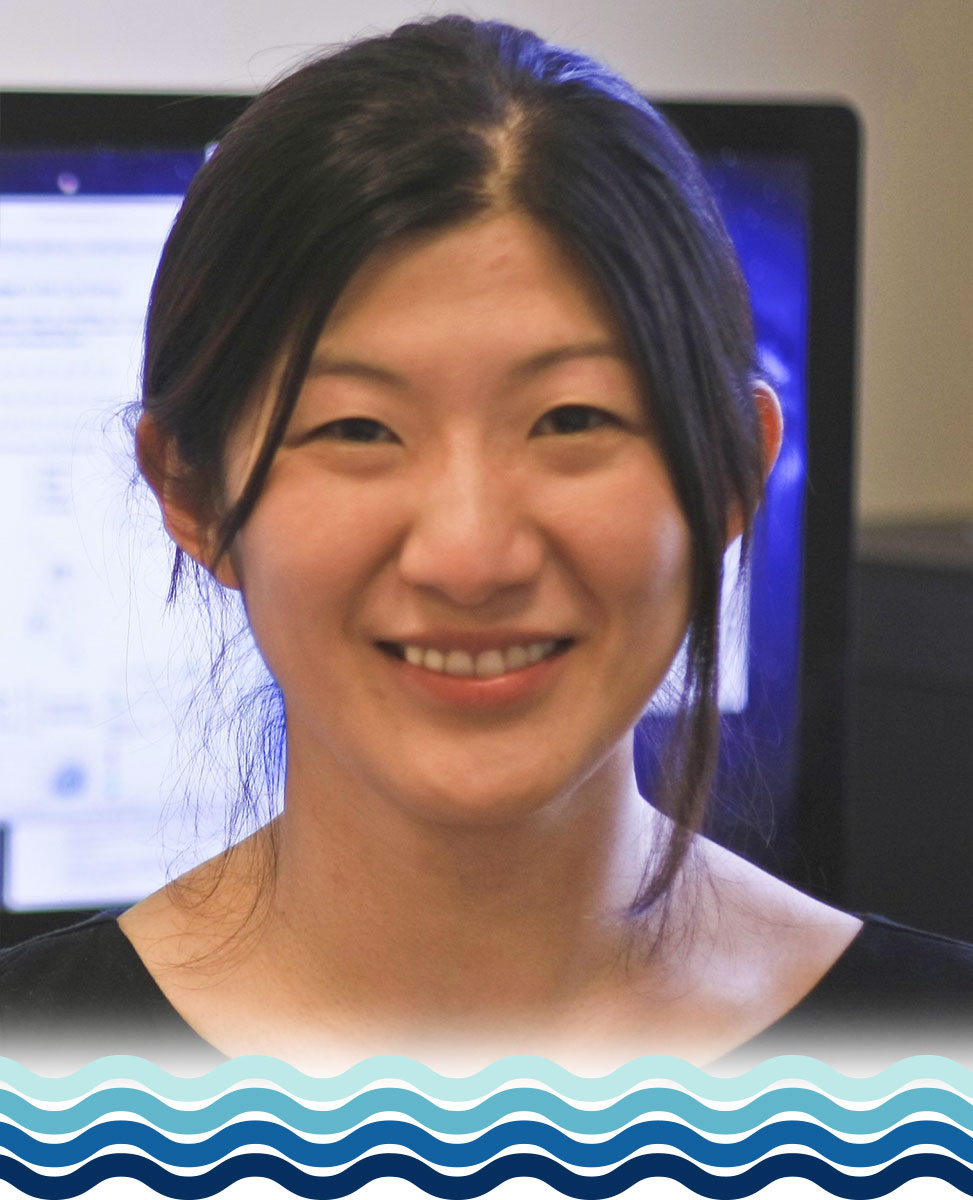
Academic Background
Yu-Shan Lin earned her B.S. in Chemistry from National Taiwan University in 2004. She completed her M.S. (2007) and Ph.D. (2009) in Chemistry at the University of Wisconsin–Madison under the mentorship of James L. Skinner. Following her doctoral studies, she was a Bio-X Postdoctoral Fellow at Stanford University from 2009 to 2012, working with Vijay S. Pande.
Professional Experience
Professor Lin joined Tufts University in 2012 as an Assistant Professor in the Department of Chemistry. She was promoted to Associate Professor in 2018 and became a full Professor and Department Chair in 2024.
Research Interests
Professor Lin's research focuses on theoretical and computational biophysical chemistry. Her group develops and applies computational methodologies to investigate biomolecular structures and functions. Key areas of interest include the design of cyclic peptides to modulate protein–protein interactions, understanding the effects of post-translational modifications and non-natural amino acids on protein folding, and studying collagen structure and stability.
Selected Honors and Awards
-
Machine Learning in the Chemical Sciences & Engineering Award, Camille and Henry Dreyfus Foundation, 2020
-
OpenEye Outstanding Junior Faculty Award in Computational Chemistry, American Chemical Society, 2015
-
Annual Women Leadership Award, Rotary Club of Cambridge, 2023
-
Rising Innovator Award, Tufts University, 2023
-
Hirschfelder Visitor, University of Wisconsin–Madison, Theoretical Chemistry Institute, 2023
Professional Activities
Professor Lin serves on the editorial advisory board for Peptide Science and is an associate editor for Biochemistry. She is also a member of the scientific advisory board for Zonsen PepLib Biotech Inc. and regularly reviews for journals such as Chemical Science, Journal of Chemical Information and Modeling, Journal of Chemical Physics, Journal of Chemical Theory and Computation, Journal of Medicinal Chemistry, Journal of Physical Chemistry B, Nature Chemistry, Physical Chemistry Chemical Physics, PLOS Computational Biology, RSC Advances, and Science.
Integrating Molecular Dynamics Simulation & Machine Learning for Cyclic Peptide Structure Prediction
A major obstacle to cyclic peptide development is that little structural information is available for these molecules, making it difficult to perform structure-based design or understand why different cyclic peptide sequences display different binding affinity, membrane permeability, and other properties. The lack of structural information is due to the fact that most cyclic peptides adopt multiple conformations in solution, existing as structural ensembles, which are very difficult to characterize using experimental techniques such as solution NMR spectroscopy.
In this talk, I will describe how we develop StrEAMM, Structural Ensembles Achieved by Molecular dynamics and Machine learning, models for cyclic peptides by combining molecular dynamics simulation and machine learning. We can now provide simulation-quality cyclic peptide structure predictions in seconds. We expect such a capability to rapidly predict cyclic peptide structures to enable researchers to understand the structural basis for the diverse properties of different cyclic peptides and greatly accelerate the development of this unique class of molecules.